Algorithm of Thought
Adversarial Reading
Bullshit is everywhere, and these algorithms of thought help you do more adversarial reading.
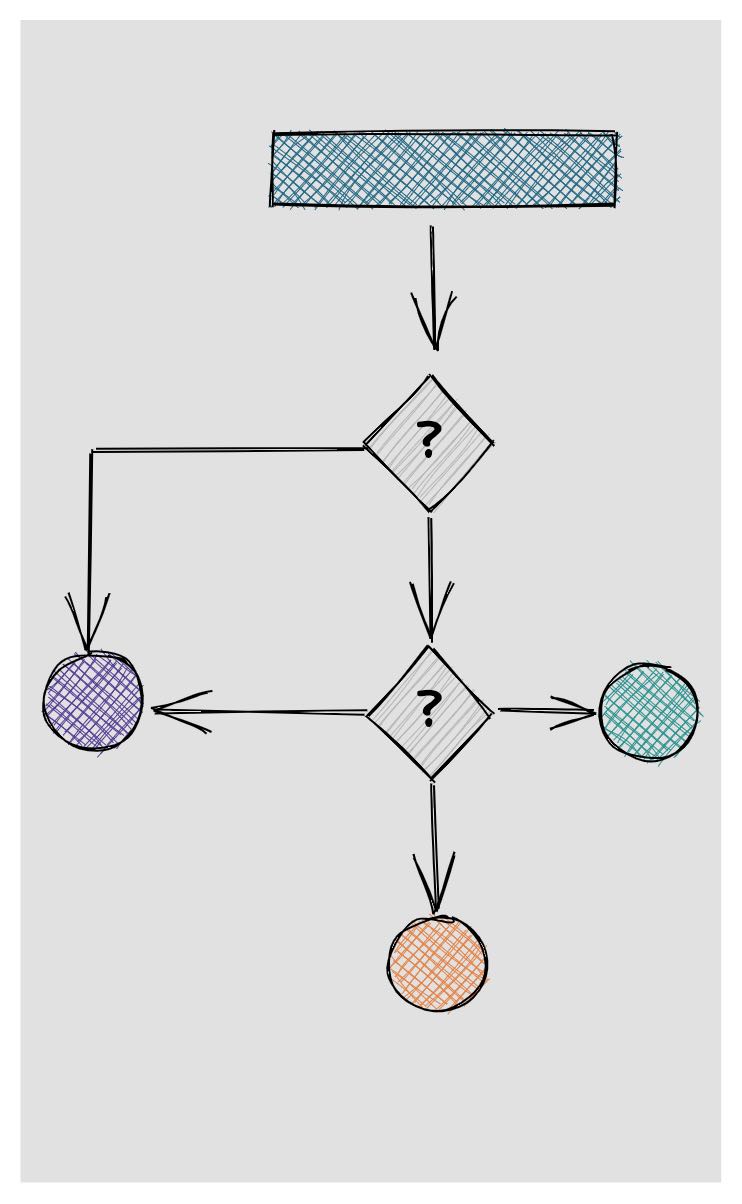
“The amount of energy necessary to refute bullshit is an order of magnitude bigger than to produce it.” ― Alberto Brandolini
Wherever we turn online to read, we're surrounded by heaps and heaps of bullshit. But even if you filter out all the toxic parts of Twitter, Reddit, and whatever else – most of the systems we live in have incentives that encourage the production of bullshit.
Eroding Trust Everywhere
Even Science is not immune: for years now the social sciences have been the scene of a replication crisis, where many of the popular lessons you've heard about psychology and other fields actually don't seem to be true.
Most often, these failures are attributed to bad methods used in the paper, publication bias, and p-hacking.
But the problems extend beyond that, and beyond the social sciences. In the opinion section of the British Medical Journal, a former editor of the journal asks whether we should "assume that health research is fraudulent until proven otherwise?".
The examples he gives aren't simple methods problems – but large numbers of outright fraudulent papers, with completely made up data. Similarly, Elisabeth Bik has identified hundreds of papers in biology that use unreliable or fake images.
So what do we do?
I've previously written about how the peer review system and academic journals are broken, and what we might do as a fix.
But that's a medium-term solution at best, and until then we still have to figure out how to navigate around the mountains of bullshit out there.
Unfortunately, there's no simple, easy way to do this – refuting bullshit takes a lot of energy, but finding it already requires vigilance. There's no way around critical thinking, which takes effort.
BUT. There is a way to make it easier, by having guidance on how to think about what we read.
Algorithms of Thought for Adversarial Reading
I've talked about Algorithms of Thought before, and the basic idea behind them is to go from the static nature of a "mental model" to something that helps you in the process of thinking.
Earning the Right To Disagree
One simple algorithm of thought comes from Adler & Van Doren's "How to Read a Book", where they discuss when you have earned the right to disagree. As the framing already suggests, however, this presumes that the source is (generally) correct and you have to defend your disagreement.
Given that most of what we read contains some amount of bullshit, however, we should look for an algorithm that is more adversarial, that helps us really probe what we're reading.
One algorithm that does this is the REAPPRAISED checklist published by Grey et al. It's not validated in any way for detecting fraudulent studies, but I'd argue it's a very solid starting point.
REAPPRAISED
The REAPPRAISED checklist consists of 58 items/questions covering research governance, ethics, authorship, productivity, plagiarism, research conduct, analyses and methods, image manipulation, statistics and data, errors, and data duplication and reporting.
Obviously, going through all 58 of these items for everything you read online is impractical. I've come up with a subset of these questions that is more manageable in our day-to-day, and generalized in a way so you can apply it to other things than scientific studies. That being said: if you read scientific studies, do check out the full checklist. I've provided a Roam template at the bottom of this post.
REALLY?
I'm calling this abbreviated checklist the REALLY? Checklist, which asks the following questions:
- Realistic Data
- Is the data collection plausible?
- Errors
- Are calculations of proportions and percentages correct?
- Are results internally consistent?
- Are the results of statistical testing internally consistent and plausible?
- Analysis
- Are the study methods plausible?
- Do the methods fit the question or claim?
- ‘P-hacking’: biased or selective analyses that promote fragile results
- Legit Statistics
- Are any data impossible or implausible?
- Are any of the outcome data unexpected outliers?
- Are there any discrepancies between reported data and (participant) inclusion criteria?
- Legible Context
- Is it clear in what context the data was collected?
- Is the result framed in the context of the data, or a broader, global context?
- Y – Why else would I doubt this?
- Anything else that gives you an uneasy feeling about this study or article?
Below, I've added this checklist and the two others as templates for Roam Research so that you can easily call them up whenever you need to work through a particular article or book.
Roam Research Templates
Right to Disagree
- Right to Disagree [[roam/templates]]
- {{[[TODO]]}} Missing information?
- {{[[TODO]]}} Wrong information?
- {{[[TODO]]}} Reasoning Errors?
- {{[[TODO]]}} Non Sequitur?
- {{[[TODO]]}} Inconsistency?
- {{[[TODO]]}} Incomplete Analysis?
REALLY?
- REALLY Checklist [[roam/templates]]
- Realistic
- {{[[TODO]]}} Is the data collection plausible?
- Errors
- {{[[TODO]]}} Are calculations of proportions and percentages correct?
- {{[[TODO]]}} Are results internally consistent?
- {{[[TODO]]}} Are the results of statistical testing internally consistent and plausible?
- Analysis
- {{[[TODO]]}} Are the study methods plausible?
- {{[[TODO]]}} Do the methods fit the question or claim?
- {{[[TODO]]}} ‘__P__-hacking’: biased or selective analyses that promote fragile results
- Legit Statistics
- {{[[TODO]]}} Are any data impossible or implausible?
- {{[[TODO]]}} Are any of the outcome data unexpected outliers?
- {{[[TODO]]}} Are there any discrepancies between reported data and (participant) inclusion criteria?
- Legible Context
- {{[[TODO]]}} Is it clear in what context the data was collected?
- {{[[TODO]]}} Is the result framed in the context of the data, or a broader, global context?
- Y – Why else would I doubt this?
- {{[[TODO]]}} Anything else that gives you an uneasy feeling about this study or article?
REAPPRAISED Checklist
- REAPPRAISED Checklist [[roam/templates]]
- ## R — Research governance
- {{[[TODO]]}} Are the locations where the research took place specified, and is this information plausible?
- {{[[TODO]]}} Is a funding source reported?
- {{[[TODO]]}} Has the study been registered?
- {{[[TODO]]}} Are details such as dates and study methods in the publication consistent with those in the registration documents?
- ## E — Ethics
- {{[[TODO]]}} Is there evidence that the work has been approved by a specific, recognized committee?
- {{[[TODO]]}} Are there any concerns about unethical practice?
- ## A — Authorship
- {{[[TODO]]}} Do all authors meet criteria for authorship?
- {{[[TODO]]}} Are contributorship statements present?
- {{[[TODO]]}} Are contributorship statements complete?
- {{[[TODO]]}} Is authorship of related papers consistent?
- {{[[TODO]]}} Can co-authors attest to the reliability of the paper?
- ## P — Productivity
- {{[[TODO]]}} Is the volume of work reported by research group plausible, including that indicated by concurrent studies from the same group?
- {{[[TODO]]}} Is the reported staffing adequate for the study conduct as reported?
- ## P — Plagiarism
- {{[[TODO]]}} Is there evidence of copied work?
- {{[[TODO]]}} Is there evidence of text recycling (cutting and pasting text between papers), including text that is inconsistent with the study?
- ## R — Research conduct
- {{[[TODO]]}} Is the recruitment of participants plausible within the stated time frame for the research?
- {{[[TODO]]}} Is the recruitment of participants plausible considering the epidemiology of the disease in the area of the study location?
- {{[[TODO]]}} Do the numbers of animals purchased and housed align with numbers in the publication?
- {{[[TODO]]}} Is the number of participant withdrawals compatible with the disease, age and timeline?
- {{[[TODO]]}} Is the number of participant deaths compatible with the disease, age and timeline?
- {{[[TODO]]}} Is the interval between study completion and manuscript submission plausible?
- {{[[TODO]]}} Could the study plausibly be completed as described?
- ## A — Analyses and methods
- {{[[TODO]]}} Are the study methods plausible, at the location specified?
- {{[[TODO]]}} Have the correct analyses been undertaken and reported?
- {{[[TODO]]}} Is there evidence of poor methodology, including:
- {{[[TODO]]}} Missing data
- {{[[TODO]]}} Inappropriate data handling
- {{[[TODO]]}} ‘__P__-hacking’: biased or selective analyses that promote fragile results
- {{[[TODO]]}} Other unacknowledged multiple statistical testing
- {{[[TODO]]}} Is there outcome switching — that is, do the analysis and discussion focus on measures other than those specified in registered analysis plans?
- ## I — Image manipulation
- {{[[TODO]]}} Is there evidence of manipulation or duplication of images?
- ## S — Statistics and data
- {{[[TODO]]}} Are any data impossible?
- {{[[TODO]]}} Are subgroup means incompatible with those for the whole cohort?
- {{[[TODO]]}} Are the reported summary data compatible with the reported range?
- {{[[TODO]]}} Are the summary outcome data identical across study groups?
- {{[[TODO]]}} Are there any discrepancies between data reported in figures, tables and text?
- {{[[TODO]]}} Are statistical test results compatible with reported data?
- {{[[TODO]]}} Are any data implausible?
- {{[[TODO]]}} Are any of the baseline data excessively similar or different between randomized groups?
- {{[[TODO]]}} Are any of the outcome data unexpected outliers?
- {{[[TODO]]}} Are the frequencies of the outcomes unusual?
- {{[[TODO]]}} Are any data outside the expected range for sex, age or disease?
- {{[[TODO]]}} Are there any discrepancies between the values for percentage and absolute change?
- {{[[TODO]]}} Are there any discrepancies between reported data and participant inclusion criteria?
- {{[[TODO]]}} Are the variances in biological variables surprisingly consistent over time?
- ## E — Errors
- {{[[TODO]]}} Are correct units reported?
- {{[[TODO]]}} Are numbers of participants correct and consistent throughout the publication?
- {{[[TODO]]}} Are calculations of proportions and percentages correct?
- {{[[TODO]]}} Are results internally consistent?
- {{[[TODO]]}} Are the results of statistical testing internally consistent and plausible?
- {{[[TODO]]}} Are other data errors present?
- {{[[TODO]]}} Are there typographical errors?
- ## D — Data duplication and reporting
- {{[[TODO]]}} Have the data been published elsewhere?
- {{[[TODO]]}} Is any duplicate reporting acknowledged or explained?
- {{[[TODO]]}} How many data are duplicate reported?
- {{[[TODO]]}} Are duplicate-reported data consistent between publications?
- {{[[TODO]]}} Are relevant methods consistent between publications?
- {{[[TODO]]}} Is there evidence of duplication of figures?